
Short Bio

Michelle Bardis is a R3 diagnostic radiology resident at the University of California Irvine School of Medicine. Her career goal is to become an innovative cardiothoracic radiologist who develops better imaging software with artificial intelligence algorithms.
In her first career, she was an electrical engineer at the NASA Jet Propulsion Laboratory and worked on the Mars Curiosity Rover that landed in 2012. She grew up in a Los Angeles suburb across the street from a commercial center whose transformation would symbolize her two careers: it was occupied by an aerospace company in her childhood and a hospital in her adulthood.
She earned her B.S. and M.S. in electrical engineering at Yale and USC respectively before obtaining her M.D. at UC Irvine. She enjoys traveling, swing dancing, and crossword puzzles.
Selected Radiology Artificial Intelligence Projects
Chest X-Rays
For this project, synthetic chest x-rays were created by generative AI programs designed by Adobe and Google by entering in descriptive text phrases. Real patient chest x-rays were gathered. Then, a deep learning network was trained to identify a synthetic versus real chest x-ray.
Kidney
For this project, patients with diseased kidneys were selected and their CTs with contrast were selected. The first neural network created a 3D box around the kidneys while a second neural network then identified the irregular contours of these kidneys. As a next step, a third neural network detected the lesions on these kidneys.
These projects were completed with three principal investigators: Dr. Peter Chang, Dr. Daniel Chow, and Dr. Roozbeh Houshyar. Special thanks for their mentorship and to UCI's Center for Artificial Intelligence in Diagnostic Medicine.
Artificial Intelligence Identifies Real vs Synthetic Chest X-Rays
Real or Synthetic?
Artificial Intelligence
Radiologist
Peer-Reviewed Publications
1. Chang, P.D., Grinband, J., Weinberg, B.D., Bardis, M.D., Khy, M., Cadena, G., Su, M.Y., Cha, S., Filippi, C.G., Bota, D., Baldi, P., Poisson, L.M., Jain, R., Chow, D.S., Deep-learning convolutional neural networks accurately classify genetic mutations in gliomas. American Journal of Neuroradiology, May 2018, DOI: https://doi.org/10.3174/ajnr.A5667, Lucien Levy Best Research Article Award for 2018
2. Shaver, M.M., Kohanteb, P.A., Chiou, C., Bardis, M.D., Chantaduly, C., Bota, D., Filippi, C.G., Weinberg, B.D., Grinband, J., Chow, D.S., Chang, P.D., Optimizing neuro-oncology imaging: A review of deep learning approaches for glioma imaging. Cancers, June 2019, DOI: https://doi.org/10.3390/cancers11060829
3. Callen, A.L., Chow, D.S., Chen, Y.A., Richelle, H.R., Pao, J., Bardis, M.D., Weinberg, B.D., Hess, C.P., Sugrue, L.P., Predictive value of noncontrast head CT with negative findings in the emergency department setting. American Journal of Neuroradiology, February 2020, DOI: https://doi.org/10.3174/ajnr.A6408
4. Glavis-Bloom, J., Houshyar, R., Ushinsky, A., Liu, H., Bui, T.L., Bardis, M.D., Fujimoto, D.K., Grant, W.A., Golshan-Momeni, M., Burns, J.E., Musculoskeletal findings on prostate MRI: Beyond metastases. Applied Radiology, March 2020.
5. Bardis, M.D., Houshyar, R., Chang, P.D., Ushinsky, A., Glavis-Bloom, J., Chahine, C., Bui, T.L., Rupasinghe, M., Filippi, C.G., Chow, D.S., Applications of artificial intelligence to prostate multiparametric MRI (mpMRI): Current and emerging trends. Urological Cancer, May 2020, DOI: https://doi.org/10.3390/cancers12051204
6. Bardis, M.D., Houshyar, R., Chantaduly, C., Ushinsky, A., Glavis-Bloom, J., Shaver, M.M., Chow, D.S., Uchio, E.M., Chang, P.D., Deep learning with limited data: Organ segmentation performance by U-Net, Electronics, July 2020, DOI: https://doi.org/10.3390/electronics9081199
7. Ushinsky, A.*, Bardis, M.D.*, Glavis-Bloom, J., Uchio, E.M., Chantaduly, C., Nguyentat, M., Chow, D.S., Chang, P.D., Houshyar, R., A 3D/2D hybrid U-Net CNN approach to prostate organ segmentation of mpMRI. American Journal of Roentgenology, January 2021, https://doi.org/10.2214/AJR.19.22168, *These authors contributed equally to this manuscript.
8. Bardis, M.D., Houshyar, R., Chantaduly, C., Tran-Harding, K., Ushinsky, A., Chahine, C., Rupasinghe, M., Chow, D.S., Chang, P.D., Prostate transition zone and peripheral zone: Segmentation with deep learning. Radiology: Imaging Cancer, In Press.
9. Houshyar, R., Glavis-Bloom, J., Bui, T., Chahine, C., Bardis, M.D., Ushinsky, A., Liu, H., Bhatter, P., Lebby, E., Fujimoto, D., Grant, W., Tran-Harding, K., Landman, J., Chow, D., Chang, P. Outcomes of Artificial Intelligence Volumetric Assessment of Kidneys and Renal Tumors for Preoperative Assessment of Nephron Sparing Interventions. Journal of Endourology, September 2021, DOI: https://doi.org/10.1089/end.2020.1125.
Other: 3 Oral Presentations at RSNA, 2 Invited Lecturer Teaching Sessions at RSNA, Multiple abstracts and posters, Reviewer for 3 journals.
Google Scholar Citations: >750
RADxx Rising Star in Medical Imaging Informatics, 2019
RSNA Medical Student Research Grant, 2019
AOA Carolyn L. Kuckein Research Fellowship, 2019
Radiology: Artificial Intelligence, Trainee Editorial Board Member, 2020-2022
Society of Interventional Oncology, AI Hackathon Runner Up 2021
Profile in RSNA News Article, 2021
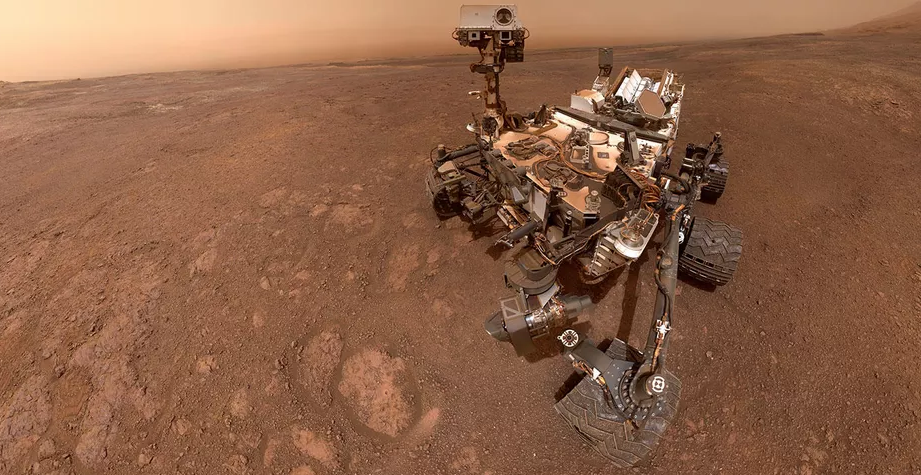
NASA Jet Propulsion Laboratory

Section 332: Communication Architecture & Research
As a graduate student, Michelle specialized in developing communication signals that send and receive data. The highlight of her career was working on the UHF signal that has brought back data from the Mars surface for 8 years on the Mars Curiosity Rover.
The UHF signal could automatically adjust its data rate from 128kbps to 2Mbps depending on the signal-to-noise ratio of the link. The UHF signal would first transmit to two orbiting satellites, Odyssey or MRO, which then relayed the signal back to one of three giant antennas on earth. Before the rover landed, its UHF signal was reproduced between buildings at JPL that served as proxies for the Mars surface and the two orbiting satellites.

